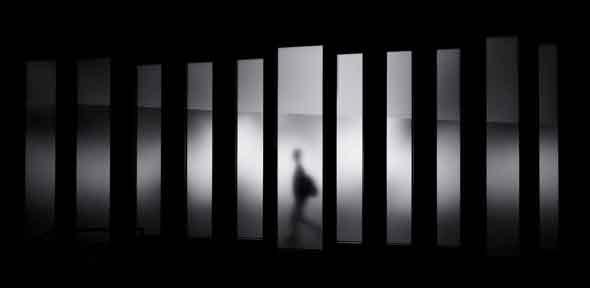
Dr Jat Singh is familiar with breaking new ground and working across disciplines. Even so, he and colleagues were pleasantly surprised by how much enthusiasm has greeted their new Strategic Research Initiative on Trustworthy Technologies, which brings together science, technology and humanities researchers from across the University.
In fact, Singh, a researcher in Cambridge’s Department of Computer Science and Technology, has been collaborating with lawyers for several years: “A legal perspective is paramount when you’re researching the technical dimensions to compliance, accountability and trust in emerging ICT; although the Computer Lab is not the usual home for lawyers, we have two joining soon.”
Governance and public trust present some of the greatest challenges in technology today. The European General Data Protection Regulation (GDPR), which comes into force this year, has brought forward debates such as whether individuals have a ‘right to an explanation’ regarding decisions made by machines, and introduces stiff penalties for breaching data protection rules. “With penalties including fines of up to 4% of global turnover or €20 million, people are realising that they need to take data protection much more seriously,” he says.
Singh is particularly interested in how data-driven systems and algorithms – including machine learning – will soon underpin and automate everything from transport networks to council services.
As we work, shop and travel, computers and mobile phones already collect, transmit and process much data about us; as the ‘Internet of Things’ continues to instrument the physical world, machines will increasingly mediate and influence our lives.
It’s a future that raises profound issues of privacy, security, safety and ultimately trust, says Singh, whose research is funded by an Engineering and Physical Sciences Research Council Fellowship: “We work on mechanisms for better transparency, control and agency in systems, so that, for instance, if I give data to someone or something, there are means for ensuring they’re doing the right things with it. We are also active in policy discussions to help better align the worlds of technology and law.”
What it means to trust machine learning systems also concerns Dr Adrian Weller. Before becoming a senior research fellow in the Department of Engineering and a Turing Fellow at The Alan Turing Institute, he spent many years working in trading for leading investment banks and hedge funds, and has seen first-hand how machine learning is changing the way we live and work.
“Not long ago, many markets were traded on exchanges by people in pits screaming and yelling,” Weller recalls. “Today, most market making and order matching is handled by computers. Automated algorithms can typically provide tighter, more responsive markets – and liquid markets are good for society.”
But cutting humans out of the loop can have unintended consequences, as the flash crash of 2010 shows. During 36 minutes on 6 May, nearly one trillion dollars were wiped off US stock markets as an unusually large sell order produced an emergent coordinated response from automated algorithms. “The flash crash was an important example illustrating that over time, as we have more AI agents operating in the real world, they may interact in ways that are hard to predict,” he says.
Algorithms are also beginning to be involved in critical decisions about our lives and liberty. In medicine, machine learning is helping diagnose diseases such as cancer and diabetic retinopathy; in US courts, algorithms are used to inform decisions about bail, sentencing and parole; and on social media and the web, our personal data and browsing history shape the news stories and advertisements we see.
How much we trust the ‘black box’ of machine learning systems, both as individuals and society, is clearly important. “There are settings, such as criminal justice, where we need to be able to ask why a system arrived at its conclusion – to check that appropriate process was followed, and to enable meaningful challenge,” says Weller. “Equally, to have effective real-world deployment of algorithmic systems, people will have to trust them.”
But even if we can lift the lid on these black boxes, how do we interpret what’s going on inside? “There are many kinds of transparency,” he explains. “A user contesting a decision needs a different kind of transparency to a developer who wants to debug a system. And a third form of transparency might be needed to ensure a system is accountable if something goes wrong, for example an accident involving a driverless car.”
If we can make them trustworthy and transparent, how can we ensure that algorithms do not discriminate unfairly against particular groups? While it might be useful for Google to advertise products it ‘thinks’ we are most likely to buy, it is more disquieting to discover the assumptions it makes based on our name or postcode.
When Latanya Sweeney, Professor of Government and Technology in Residence at Harvard University, tried to track down one of her academic papers by Googling her name, she was shocked to be presented with ads suggesting that she had been arrested. After much research, she discovered that “black-sounding” names were 25% more likely to result in the delivery of this kind of advertising.
Like Sweeney, Weller is both disturbed and intrigued by examples of machine-learned discrimination. “It’s a worry,” he acknowledges. “And people sometimes stop there – they assume it’s a case of garbage in, garbage out, end of story. In fact, it’s just the beginning, because we’re developing techniques that can automatically detect and remove some forms of bias.”
Transparency, reliability and trustworthiness are at the core of Weller’s work at the Leverhulme Centre for the Future of Intelligence and The Alan Turing Institute. His project grapples with how to make machine-learning decisions interpretable, develop new ways to ensure that AI systems perform well in real-world settings, and examine whether empathy is possible – or desirable – in AI.
Machine learning systems are here to stay. Whether they are a force for good rather than a source of division and discrimination depends partly on researchers such as Singh and Weller. The stakes are high, but so are the opportunities. Universities have a vital role to play, both as critic and conscience of society. Academics can help society imagine what lies ahead and decide what we want from machine learning – and what it would be wise to guard against.
Weller believes the future of work is a huge issue: “Many jobs will be substantially altered if not replaced by machines in coming decades. We need to think about how to deal with these big changes.”And academics must keep talking as well as thinking. “We’re grappling with pressing and important issues,” he concludes. “As technical experts we need to engage with society and talk about what we’re doing so that policy makers can try to work towards policy that’s technically and legally sensible.”
Fairness, trust and transparency are qualities we usually associate with organisations or individuals. Today, these attributes might also apply to algorithms. As machine learning systems become more complex and pervasive, Cambridge researchers believe it’s time for new thinking about new technology.
The text in this work is licensed under a Creative Commons Attribution 4.0 International License. For image use please see separate credits above.